- Interpreting Quantitative Trading Models and Comprehending Quantitative Trading
- Backdating's Function in Quantitative Trading
- Statistical Risk Analysis
- The Role of AI and Machine Learning in Quantitative Trading in the Future
- Quantitative Trading's Morals
- Quantitative Trading's Application in Various Financial Markets
- Quantitative Trading's Human Factor
- Conclusion
A thorough understanding of both finance and programming concepts is necessary to write an assignment on quantitative trading strategies. Algorithmic design, backtesting, risk management, and strategy evaluation are just a few of the topics you'll explore. We'll give you the knowledge and insights you need to succeed in your coursework throughout this guide.
This guide will give you the tools and methods to write an extensive assignment, whether you're a finance student or a professional looking to improve your abilities. Statistical analysis, time series modeling, machine learning algorithms, and other key elements of quantitative trading strategies will all be covered. You'll learn about the difficulties faced by traders, the value of thorough backtesting, and how risk management functions in quant strategies.
You'll have a strong foundation by the end of this guide to build a well-organized and educational assignment on quantitative trading strategies. Together, let's set out on this adventure to discover the mysteries of this fascinating field.
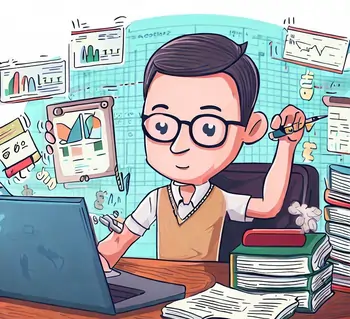
Interpreting Quantitative Trading Models and Comprehending Quantitative Trading
Let's start by developing a thorough understanding of the subject at hand before we get into the specifics of how to structure your assignment. The core of quantitative trading is the creation of trading strategies that are executed using fast computers and sophisticated mathematical models. These tactics rely on patterns that are derived from the mathematical interpretation of market data and are data-dependent.
Numerous elements can be included in a quantitative trading strategy, from understanding market volatility and risk management to forecasting future price movements. Traders create algorithms that forecast future changes in stock prices using statistical and computational methods. The real-time trading market is then used to execute trades using these algorithms.
Understanding quantitative trading strategies begins with interpreting quantitative trading models. Mean Reversion, Momentum, Statistical Arbitrage, and Machine Learning models are just a few of the well-known models used by quant traders.
Strategies based on mean reversion make the assumption that prices and returns will eventually return to the mean or average. This is the fundamental idea behind trading strategies like pair trading, which involves pairing up two historically correlated securities with the expectation that one will move first and the other will follow.
Conversely, momentum strategies adhere to the maxim "the trend is your friend." Here, traders attempt to capitalize on a stock's momentum by going long or short in the expectation that the price will move further in that direction.
Statistical arbitrage strategies are high-frequency trading techniques that take advantage of relative mispricing between securities using statistical and econometric techniques.
Last but not least, machine learning strategies employ computational algorithms that develop and improve based on data, making forecasts or judgments without being explicitly programmed.
Backdating's Function in Quantitative Trading
Now that you have a firm grasp of quantitative trading and its various models, let's look at backdating, which is an essential step in the procedure. Backdating is the process of using historical data to evaluate the performance of a strategy. It serves as the yardstick for any quantitative trading strategy and is crucial to the validation and improvement of any model.
However, you must take into account some of the backtesting's pitfalls, such as curve fitting and overfitting, when writing about it. Curve fitting is the process of creating a model that is specifically designed to fit historical data perfectly but may struggle with new data. When a model has too many parameters in relation to the number of observations, it is said to be overfit. The model's predictive ability is compromised in both scenarios.
Statistical Risk Analysis
Quantitative trading involves both the identification of potential opportunities and the management of associated risks. A thorough understanding of statistics, financial theory, and computational algorithms are necessary for risk management in quantitative trading, which is a broad and complex field.
The steps in the quantitative risk management process include locating the risk's source, estimating its magnitude, and making management choices. Risk measurement, which makes use of models like Value at Risk (VaR), Conditional Value at Risk (CVaR), and Stress Testing, is a significant component of risk management.
Value at Risk (VaR) is a term used to describe the potential decline in value of a risky asset or portfolio over a specified time period for a given confidence interval. The risk assessment metric CVaR, also known as Expected Shortfall (ES), measures the likelihood of extreme losses in the tail of a distribution of potential returns. On the other hand, stress testing involves estimating potential losses under extreme but realistic scenarios.
The Role of AI and Machine Learning in Quantitative Trading in the Future
Understanding the function of AI and machine learning is crucial as we move into the future of quantitative trading. Quantitative trading has been transformed by these cutting-edge technologies, which offer unmatched predictive capabilities, risk management, and decision-making support.
Massive amounts of data can be processed by AI and machine learning at breakneck speeds, and these systems can then use the data to continuously improve their forecasts. This quality is particularly advantageous in quantitative trading because daily data production on financial markets is enormous.
Additionally, Deep Learning, a branch of machine learning, is causing a stir in the world of quantitative trading. Deep learning algorithms continually examine data using a predetermined logical structure in an effort to reach conclusions that are comparable to those reached by humans. An artificial neural network (ANN) is a layered structure of algorithms that they employ to accomplish this. An ANN's design is influenced by how the human brain works biologically, opening the door to much more complex and nuanced data interpretation.
Quantitative Trading's Morals
It's important to take into account the ethical ramifications of quantitative trading while exploring the topic further. Concerns about market manipulation, flash crashes, and even wider economic instability have been raised because these strategies are primarily algorithm-based.
If high-frequency trading (HFT) algorithms are created to artificially inflate or deflate prices for profit, market manipulation may take place. A case in point is "spoofing," in which an algorithm generates fictitious market orders in an effort to deceive other participants and profit from the ensuing price changes.
Another important ethical issue is flash crashes. The most well-known instance is the incident on May 6, 2010, when the Dow Jones Industrial Average dropped by about 1000 points before quickly making up those losses. Many believe that this "Flash Crash" was caused by HFT algorithms responding to particular market circumstances.
A larger economic worry is that by accelerating price changes and market correlations, quantitative trading can exacerbate market bubbles and crashes.
Therefore, while completing your assignment, think about the ethical implications for society and the societal implications of quantitative trading. Also, evaluate the steps taken to address these issues and possible solutions for a more stable and equitable market.
Quantitative Trading's Application in Various Financial Markets
Investigating the use of quantitative trading strategies on various financial markets is also beneficial. Quantitative trading strategies are used on a variety of financial markets, including forex, commodities, and derivatives, despite being frequently associated with stock markets.
The foreign exchange (forex) market is ideally suited for algorithmic trading due to its high liquidity and 24-hour trading cycle. In forex trading, methods like Statistical Arbitrage and Market Making are frequently employed.
Similar to this, algorithms are used in the commodity markets to forecast price changes based on variables like weather patterns, geopolitical developments, and changes in supply and demand. Options pricing models like the Black-Scholes-Merton and Binomial models are frequently used in algorithmic trading strategies in the derivatives market.
Quantitative Trading's Human Factor
Last but not least, remember that quantitative trading involves people. While it is simple to become engrossed in the intricate algorithms and mathematical models, keep in mind that a team of quants, developers, and traders stands behind every strategy. These strategies were developed and are successful thanks to their knowledge, originality, and critical thinking.
Additionally, the strategies are reflections of how people behave. They are intended to spot recurring patterns and tendencies brought about by widespread human behavior. Understanding this can give your assignment a new perspective and depth.
Writing an essay on quantitative trading strategies can be challenging, but if you grasp the fundamental ideas and subtleties of the subject and critically evaluate its wider implications and applications, you can produce a thorough and insightful essay. Remember to keep your explanation and analysis simple, back up your claims with examples from real life, and—most importantly—present a fair assessment of the advantages and disadvantages that quantitative trading offers.
Conclusion
In conclusion, a thorough understanding of financial, programming, and algorithmic concepts is necessary to write an assignment on quantitative trading strategies. We have looked at the crucial elements and subtleties of creating a thorough assignment in this field throughout this guide. The foundations of quantitative trading strategies and the significance of data-driven decision-making in the financial markets were first understood. We talked about the various difficulties that traders face and emphasized the value of thorough backdating and risk management in the creation and assessment of quant strategies. The importance of statistical analysis, time series modeling, machine learning algorithms, and their use in quantitative trading was also covered in-depth. You'll be better able to analyze financial data, spot patterns, and create trading strategies based on reliable quantitative principles if you gain knowledge in these areas. In addition, we highlighted John Smith's credentials and experience in quantitative trading strategies. He is a recognized authority in the area. As you work on your assignment or research career options in this field, these experts can offer insightful advice. Not only must you present information in a quantitative trading strategies assignment, but you must also show that you can think critically and apply theoretical ideas to real-world situations. You can create an engaging and educational assignment by adhering to the rules and comprehending the fundamental concepts covered in this manual. It takes time, practice, and a strong background in finance and programming to master the art of writing an assignment on quantitative trading strategies. To advance your knowledge and succeed in your academic or professional endeavors, embrace the challenges, maintain your curiosity, and continue to research this fascinating field.