- Evaluating Correlation Coefficient Significance
- Comparing Correlation Coefficients
- Understanding Residuals
- Predicting Beyond Data Range
- Interpreting the Slope
- Creating and Using Linear Models
- Predicting with Linear Models
- Prediction Intervals
- R Commands for Regression Analysis
- Conclusion
In the realm of statistical analysis, particularly when dealing with regression analysis, understanding the significance and relevance of correlation coefficients is crucial. This is especially true when working with constrained or limited data sets. This blog delves into the nuances of correlation coefficients in small samples and their implications for linear models.
Understanding the relevance of correlation coefficients in constrained data sets is crucial for accurate data analysis. Correlation coefficients measure the strength and direction of the linear relationship between two variables. In small or limited data sets, interpreting these coefficients can be challenging, as even a high correlation might not always indicate statistical significance. Analyzing residuals and ensuring predictions are within the data range are key aspects of effective regression analysis.
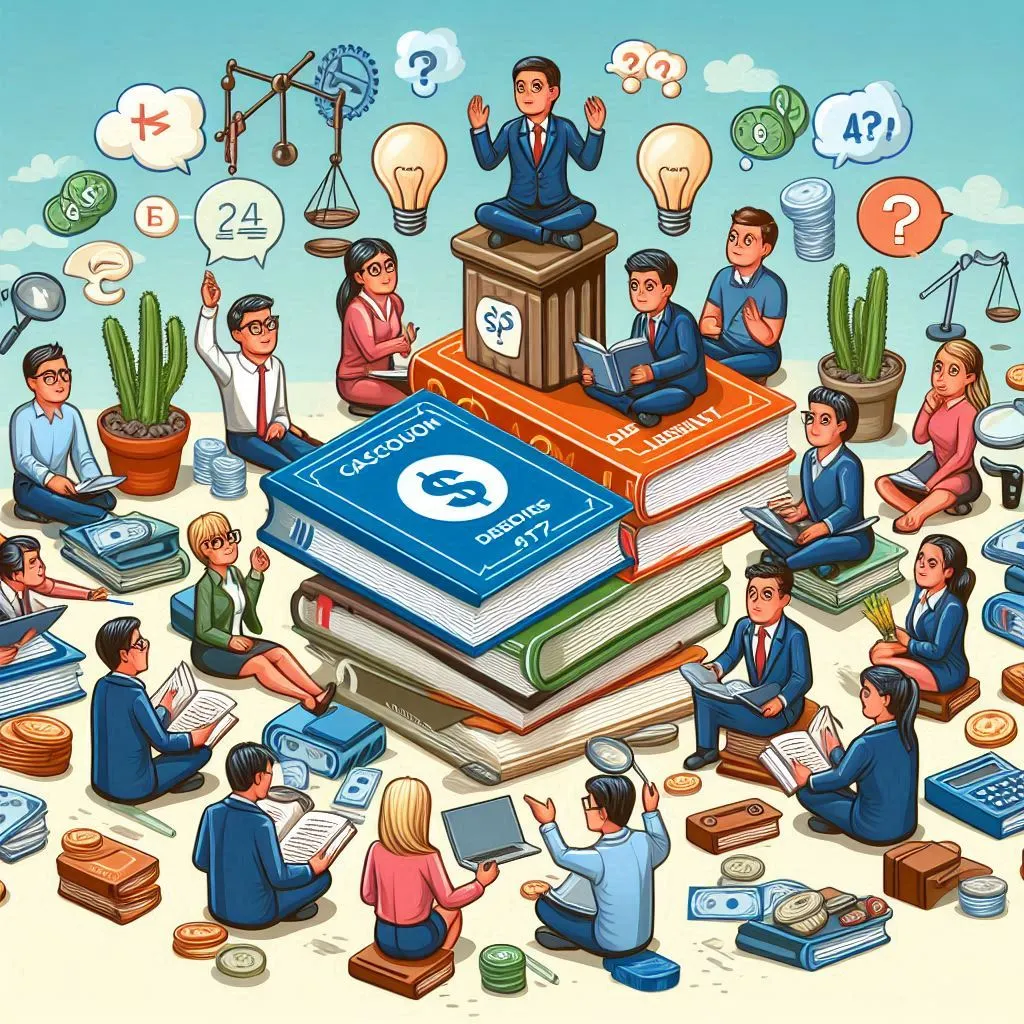
For students working on regression analysis assignments, discerning the significance of these coefficients and applying correct statistical methods can be complex. In such cases, assistance with Regression Analysis assignments can provide valuable support. Expert help can guide you through evaluating correlation coefficients, interpreting residuals, and developing reliable models based on your data. Utilizing professional assistance ensures a thorough understanding of these concepts and enhances the accuracy of your analysis.
Evaluating Correlation Coefficient Significance
Evaluating Correlation Coefficient Significance in Limited Data Contexts is an essential step in statistical analysis. When you have a small data set, the significance of a correlation coefficient can be challenging to determine accurately. In small samples, even a seemingly strong correlation might not be statistically significant due to the limited number of observations. For example, a correlation coefficient of 0.58 in a data set of just 10 pairs of (x, y) data points suggests a moderate relationship, but whether this is statistically significant requires a more detailed analysis, considering the sample size and the level of significance.
Comparing Correlation Coefficients
When comparing two correlation coefficients, such as 0.83 and 0.45, the higher value (0.83) represents a stronger linear relationship. In the context of small data sets, understanding which coefficient indicates a stronger relationship helps in making informed decisions about the data's underlying patterns. The larger the correlation coefficient, the stronger the linear relationship between the variables.
Understanding Residuals
Residuals play a pivotal role in evaluating the accuracy of a regression model. For a given data point where the predicted y-value is 22.7 and the actual y-value is 25.4, the residual is positive (2.7). Residuals are calculated as the difference between the observed value and the predicted value, providing insights into the model's accuracy and how well it fits the data. Positive residuals indicate that the model has under-predicted the actual value, while negative residuals suggest over-prediction.
Predicting Beyond Data Range
When applying a linear regression model developed with data ranging from 1 to 15 years of experience to predict salaries for someone with 25 years of experience, caution is warranted. Predictions made outside the range of the data used to develop the model can be unreliable. Such extrapolations might not accurately reflect the true relationship and should be approached with skepticism.
Interpreting the Slope
The slope of a linear regression model represents the change in the dependent variable (y) for every unit increase in the independent variable (x). For instance, if the slope indicates that for each additional year of experience, the salary increases by $2000, this is a direct measure of how changes in one variable affect the other. The slope thus provides critical insights into the strength and direction of the relationship between the variables.
Creating and Using Linear Models
To develop a linear regression model, you should first create a scatter plot to visualize the data. Following this, calculate the correlation coefficient to assess the strength of the relationship. Check for significance to ensure the relationship is statistically meaningful, and then create the linear model based on these analyses. This sequence ensures that the model you develop is robust and reliable.
Predicting with Linear Models
For a linear model used to predict outcomes such as salaries based on years of experience, the model’s equation and correlation strength are crucial. If the model indicates a significant correlation, you can use it to make predictions, but always consider the limitations and range of the data used.
Prediction Intervals
As you make predictions for x-values that are further from the mean of x, the prediction intervals typically become larger. This is because the certainty of predictions decreases as you move further from the observed data range. Hence, predictions for extreme values should be made with caution.
R Commands for Regression Analysis
In R, the command cor is used to calculate the correlation coefficient, while lm is used to compute the slope and y-intercept for linear regression models. Mastery of these commands is essential for accurate data analysis and model creation.
Conclusion
Discerning the relevance of correlation coefficients in constrained data sets requires a nuanced understanding of statistical principles and practical application. By carefully evaluating significance, comparing coefficients, understanding residuals, and making cautious predictions, you can effectively analyze and interpret your data. These skills are crucial for developing reliable models and making informed decisions based on statistical analysis. For those seeking to enhance their understanding or tackle challenging problems, finance assignment help can be invaluable. Expert assistance can guide you through the intricacies of correlation analysis, ensuring a clear grasp of how to apply these concepts in your assignments.